Summary Tables
Overview
Aggregating data before it's queried can decrease query latency and reduce system load. Hydrolix supports aggregation via summary tables. You can use summary tables to store aggregations including minimum, maximum, count, and sum. In certain cases, summary tables can store statistical aggregations like unique counts and quantiles/percentiles.
When you use summary tables, Hydrolix aggregates data with the following steps:
- Hydrolix runs a summary SQL transform on raw table partitions to produce intermediate aggregation data.
- Hydrolix writes the output of that SQL statement to a summary table.
Later, queries can use the intermediate aggregation data stored in the summary table to process aggregation queries faster than they could by processing every individual piece of input data.
The series of transforms that intake peers apply to raw data.
More than one summary can exist on a parent table. For example, the diagram below shows a table that provides raw data for both minute and hourly summary tables:
Two summary tables are simultaneously produced from one parent table.
Create the Summary Transform
The summary transform defines a SQL statement that aggregates your data over some period of time.
Summary Transform Structure
The following example shows a general form for summary transforms:
SELECT
<time_field_aggregation> AS <alias>,
<function_on_column> AS <alias>,
.......
FROM
<parent_project.parent_table>
GROUP BY
<time_field_alias>
SETTINGS
hdx_primary_key = '<time_field_alias>'
Summary transforms are subject to the following limitations:
- In the
SETTINGS
clause, you must specify ahdx_primary_key
. This primary key should correspond to a time field aggregation alias in yourSELECT
statement, and it will be used to signal which aliased column in your query is being used as the primary key in your summary table. - In the
FROM
clause, specify the project name and the table name of the parent table.
Example Summary Transform
Consider a summary table that aggregates, on an minute-by-minute basis, for an e-commerce site:
- the sum of item cost
- the average tax
- the 95th quantile of distance
The following SQL shows how you could translate these requirements into a summary transform:
select
toStartOfMinute(timestamp) as minute,
sum(cost) as sumcost,
avg(tax) as avgtax,
quantile(0.95)(distance) as _95th
from project.parent_table
group by minute
settings hdx_primary_key='minute'
When constructing a summary transform, the API will reject any non-deterministic summary SQL clauses.
Functions now()
, today()
, or current_timestamp()
are not accepted in a WHERE clause for a summary SQL transform. This would introduce data not present in the underlying table. It is safe to operate on timestamps as shown above with toStartofMinute(timestamp)
or even performing math using INTERVAL
.
Window functions, like PARTITION BY
or OVER
are also considered non-deterministic in the context of summary transform execution. They rely on access to all underlying data within the requested window, which is not guaranteed given the distributed partitions. Window functions are rejected in summary SQL clauses.
Exclude columns from indexing
A summary table indexes all non-aggregate columns by default.
Add this line to the transform SQL query to exclude columns from indexing:
hdx_summary_override_indexes = 'column_1,column_2,column_3'
In this example, the sumcost
and avgtax
columns are not indexed.
SELECT
toStartOfMinute(timestamp) AS minute,
SUM(cost) AS sumcost,
AVG(tax) AS avgtax,
QUANTILE(0.95)(distance) as _95th
FROM project.parent_table
GROUP BY minute
SETTINGS hdx_primary_key = 'minute',
hdx_summary_override_indexes = 'sumcost,avgtax'
Create the Summary Table
Defining a summary table includes the transform above. It can be created, modified, and deleted in Hydrolix by using the Hydrolix UI or via the table management methods in the config API.
Via the UI
To create a summary table with the Hydrolix UI:
- In the top right of the Hydrolix UI, click the "+ Add New" button.
- From the options in the right sidebar, select "Summary Table". You will see this form:
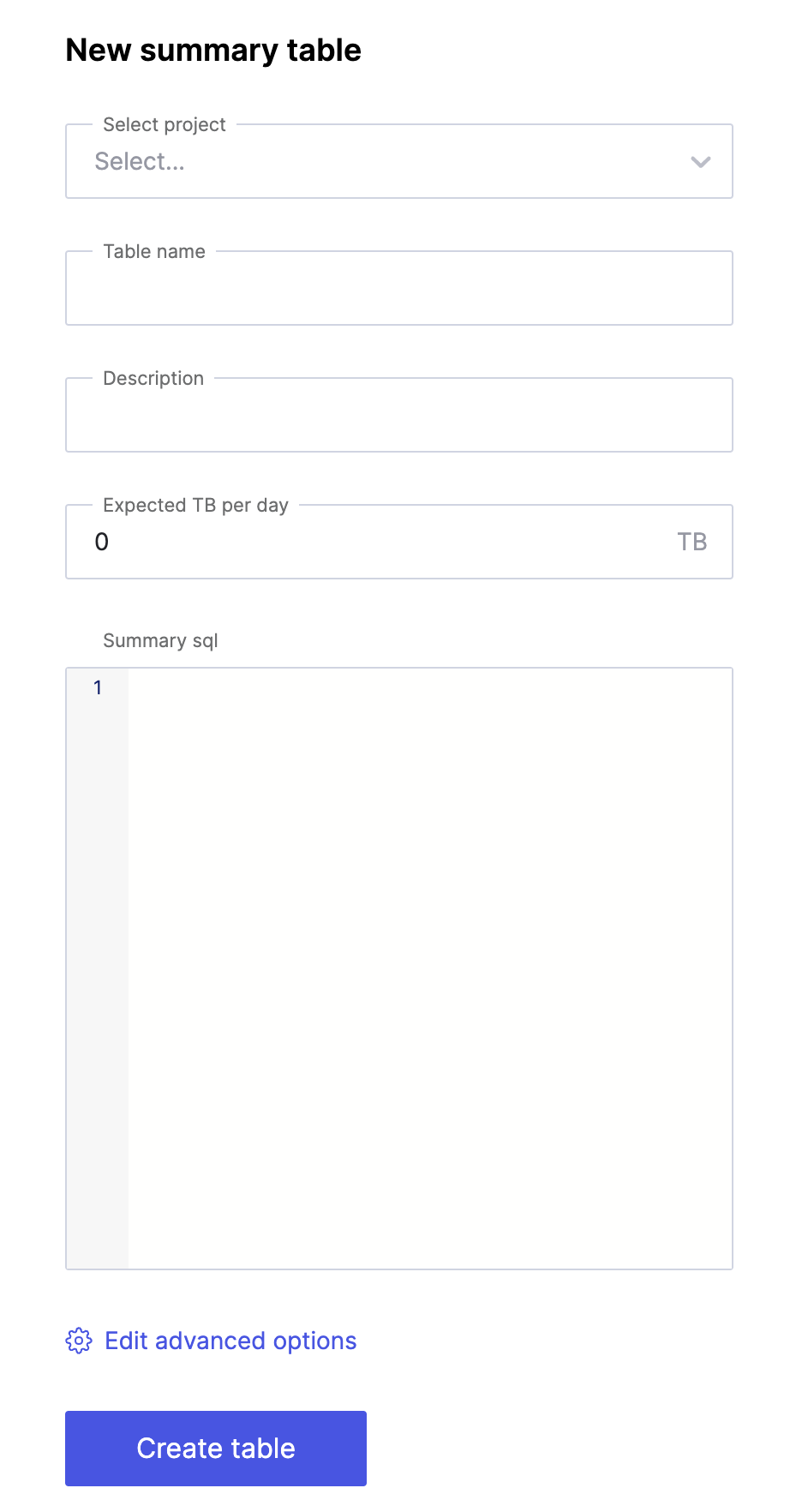
Creating a summary table in the Hydrolix UI.
- Specify the following:
- a project where you wish to place the table
- a table name
- a description for the table
- In the "Summary sql" field, paste the summary transform you defined above. Don't forget the
SETTINGS
clause. - Click "Create table" to create your table.
Via the API
After logging into the API to receive the bearer token, create a table with the summary enabled and your summary transform.
Log Into to the API
Use your favorite HTTP API tool to log into the API to receive your Bearer token. If you are using cURL, for example:
Get the bearer token, which is good for the next 24 hours, to authenticate future API calls. This command assumes you've set the $HDX_HOSTNAME, $HDX_USER and $HDX_PASSWORD environment variables:
export HDX_TOKEN=$(
curl -v -X POST -H "Content-Type: application/json" \
https://$HDX_HOSTNAME/config/v1/login/ \
-d "{
\"username\":\"$HDX_USER\",
\"password\":\"$HDX_PASSWORD\"
}" | jq -r ".auth_token.access_token"
)
Create the Table
Using the same tool as above (or your favorite API tool), send a POST
request to the table creation endpoint at /config/v1/orgs/{org_id}/projects/{project_id}/tables/
.
{
"name": "my.summarytable",
"description": "Minute-by-minute summary of parent table",
"type": "summary",
"settings": {
"merge": {
"enabled": true
},
"summary": {
"enabled": true,
"sql": "select\n toStartOfMinute(timestamp) as minute,\n sum(cost) as sumcost,\n avg(tax) as avgtax,\n quantile(0.95)(distance) as _95th\nfrom project.parent_table\ngroup by minute\nsettings hdx_primary_key='minute'"
}
}
}
curl --request POST \
--url https://$HDX_HOSTNAME/config/v1/orgs/$HDX_ORG/projects/$HDX_PROJECT/tables/ \
--header "Authorization: Bearer $HDX_TOKEN" \
--header "Accept: application/json" \
--header "Content-Type: application/json" \
--data "
{\"name\": \"my.summarytable\",
\"description\": \"Minute-by-minute summary of parent table\",
\"type\": \"summary\",
\"settings\": {
\"merge\": {
\"enabled\": true
},
\"summary\": {
\"enabled\": true,
\"sql\": \"select\n toStartOfMinute(timestamp) as minute,\n sum(cost) as sumcost,\n avg(tax) as avgtax,\n quantile(0.95)(distance) as _95th\nfrom project.parent_table\ngroup by minute\nsettings hdx_primary_key='minute'\"
}
}
}
"
{
"project": "{project_id}",
"name": "my.summarytable",
"description": "Minute-by-minute summary of parent table",
"uuid": "68af42f6-5479-4b07-b873-4c8281d8432a",
"created": "2024-02-29T20:02:11.533434Z",
"modified": "2024-02-29T20:02:15.423424Z",
"settings": {
"summary": {
"sql": "select\n toStartOfMinute(timestamp) as minute,\n sum(cost) as sumcost,\n avg(tax) as avgtax,\n quantile(0.95)(distance) as _95th\nfrom project.parent_table\ngroup by minute\nsettings hdx_primary_key='minute'",
"enabled": true,
"parent_table": "20f23a3f-d2d5-4657-87d3-0a99ed56bd6c"
},
"stream": {
"token_list": [],
"hot_data_max_age_minutes": 60,
"hot_data_max_active_partitions": 12,
"hot_data_max_rows_per_partition": 1048576,
"hot_data_max_minutes_per_partition": 5,
"hot_data_max_open_seconds": 10,
"hot_data_max_idle_seconds": 5,
"cold_data_max_age_days": 3650,
"cold_data_max_active_partitions": 168,
"cold_data_max_rows_per_partition": 1048576,
"cold_data_max_minutes_per_partition": 30,
"cold_data_max_open_seconds": 60,
"cold_data_max_idle_seconds": 30
},
"age": {
"max_age_days": 0
},
"reaper": {
"max_age_days": 1
},
"merge": {
"enabled": true,
"sql": "SELECT `toStartOfMinute(timestamp)`, _CAST(sumMergeState(_CAST(`sum(cost)`, 'AggregateFunction(sum, Nullable(Float64))')), 'String') AS `sum(cost)`, _CAST(avgMergeState(_CAST(`avg(tax)`, 'AggregateFunction(avg, Nullable(Float64))')), 'String') AS `avg(tax)`, _CAST(quantileMergeState(0.95)(_CAST(`quantile(0.95)(distance)`, 'AggregateFunction(quantile(0.95), Nullable(Float64))')), 'String') AS `quantile(0.95)(distance)` FROM `{STREAM}` GROUP BY `toStartOfMinute(timestamp)` FORMAT HDX SETTINGS hdx_primary_key = 'minute', optimize_fuse_sum_count_avg = 1, optimize_syntax_fuse_functions = 1, hdx_format_json_schema = '[{\"datatype\":{\"format\":\"%Y-%m-%d %H:%M:%S\",\"index\":false,\"primary\":true,\"type\":\"datetime\"},\"name\":\"toStartOfMinute(timestamp)\"},{\"datatype\":{\"denullify\":true,\"index\":false,\"type\":\"string\"},\"name\":\"sum(cost)\"},{\"datatype\":{\"denullify\":true,\"index\":false,\"type\":\"string\"},\"name\":\"avg(tax)\"},{\"datatype\":{\"denullify\":true,\"index\":false,\"type\":\"string\"},\"name\":\"quantile(0.95)(distance)\"}]'"
},
"autoingest": [
{
"enabled": false,
"source": "",
"pattern": "",
"max_rows_per_partition": 12288000,
"max_minutes_per_partition": 60,
"max_active_partitions": 50,
"dry_run": false,
"transform": "52f65fa7-817f-4aaa-aa9f-80201c8188da"
}
],
"sort_keys": [],
"shard_key": null,
"max_future_days": 0,
"max_request_bytes": 0
},
"url": "https://{hostname}/config/v1/orgs/{org_id}/projects/{project_id}/tables/68af42f6-5479-4b07-b873-4c8281d8432a",
"type": "summary",
"primary_key": "toStartOfMinute(timestamp)"
}
Query the Summary Table
Use the aliases specified in your summary SQL to query your data.
Don't use
SELECT *
This will return the internal, intermediate forms of summary data. Instead, include each alias name that you want the query to return.
For instance, the following example uses aliases specified in the example above:
select
minute,
sumcost,
avgtax,
_95th
from my.summary_table
where minute between '2023-01-01 00:00:00' and '2023-01-02 00:00:00'
group by minute
order by minute
To learn more about the aliases in your summary table, see Describe the Schema below.
Group by
is essentialYou must specify a
group by
containing a subset of the columns used in thegroup by
clause of the summary transform.
Additional Considerations
Merge peers, intake-heads, and indexers
When combined with summary-based dashboards and other queries, summary tables can significantly decrease the query load on your Hydrolix cluster. However, summary tables can increase the workload of intake peers, merge peers, and the indexer, so keep this in mind when adding them. Monitor your cluster's pods when turning summaries on so you're aware of shifting load and costs in your cluster.
For more information about scaling, see the Scaling your Cluster page.
Permissions
RBAC considers summary tables to be like other tables, so users will need these permissions to edit, modify, and delete summary tables:
view_table
add_table
change_table
delete_table
In a default Hydrolix installation, users with the super_admin
role have these permissions. See the User Permissions (RBAC) documentation for more information.
Batch Ingest
As of Hydrolix version 4.14, summary tables using data from the tables in which you load data will also be populated during batch ingest.
Aggregates of Aggregates
Hydrolix stores summary data in intermediate formats. These intermediate formats don't just store an aggregation result; they also store supplemental data that enables accurate calculation of additional aggregations.
For example, you normally can't calculate a weighted average of two averages. But Hydrolix's intermediate formats also store weighting data for averages, so you can aggregate already-calculated averages.
As a result, you can aggregate the aggregate data stored in your summary tables. The above example stores intermediate data in one-minute segments. Intermediate states enable an hourly aggregation of those minute-long segments:
select toStartOfHour(time) as hour,
sumcost,
avgtax,
_95th
from
( select
minute,
sumcost,
avgtax,
_95th
from my.summarytable
where time between '2023-01-01 00:00:00' and '2023-01-02 00:00:00'
group by minute
)
group by hour
order by hour
Describe the Schema
To make summary data simpler to work with, Hydrolix automatically creates alias names for common aggregation functions. To see a full list of generated aliases, execute a DESCRIBE
query on your table:
DESCRIBE TABLE my.summarytable
Query id: 732ac0b2-c6f8-46cd-be29-edfabccdcdd6
┌─name──────────────────────────────────────────────────┬─type─────────────────────────────────────────────────┬─default_type─┬─default_expression────────────────────────────────────────────────────────┬─comment─┬─codec_expression─┬─ttl_expression─┐
│ toStartOfFiveMinute(time) │ DateTime │ │ │ │ │ │
│ sum(sumcost) │ AggregateFunction(sum, Nullable(UInt64)) │ │ │ │ │ │
│ avg(avgtax) │ AggregateFunction(avg, Nullable(Int32)) │ │ │ │ │ │
│ quantile(0.95)(arrayElement(map_double, 'double')) │ AggregateFunction(quantile(0.95), Nullable(double)) │ │ │ │ │ │
│ time │ DateTime │ ALIAS │ `toStartOfFiveMinute(timestamp)` │ │ │ │
│ sumcost │ Nullable(UInt64) │ ALIAS │ sumMerge(`sum(sumcost)`) │ │ │ │
│ avgtax │ Nullable(Float64) │ ALIAS │ avgMerge(`avg(avgtax)`) │ │ │ │
│ _95th │ Nullable(Float64) │ ALIAS │ quantileMerge(0.95)(`quantile(0.95)(arrayElement(map_double, 'double'))`) │ │ │ │
└───────────────────────────────────────────────────────┴──────────────────────────────────────────────────────┴──────────────┴───────────────────────────────────────────────────────────────────────────┴─────────┴──────────────────┴────────────────┘
[query-head-64bcccdcc5-qk2km] 2023.01.30 18:29:33.255360 [ 9 ] {732ac0b2-c6f8-46cd-be29-edfabccdcdd6} <Information> executeQuery: Read 8 rows, 1.07 KiB in 0.00077993 sec., 10257 rows/sec., 1.33 MiB/sec.
8 rows in set. Elapsed: 0.271 sec.
query-peer :)
Updated 2 days ago